Initiative details
Valerann is tackling critical road safety challenges associated with complexity and criticality of road traffic management and road safety.
Traditional road monitoring systems often rely heavily on fixed infrastructure, which can be limited in coverage and responsiveness. The increase of data sources (CAV, crowdsourced data, navigation apps etc) brings an opportunity to enrich situational awareness of TMCs; but it also brings a challenge of data fragmentation, data overload and confusion as deliver high volumes of unstructured data and false positives, overwhelming operators and delaying meaningful action. In response, Valerann deployed Lanternn by Valerann™, an AI-powered data fusion platform that transforms how traffic data is collected, processed, and acted upon. The key safety challenge being addressed is the speed and accuracy of incident detection. Delays in identifying road incidents can increase the risk of secondary collisions, congestion, and response inefficiency. LbV synthesises diverse data sources—floating car data, roadside sensors, navigation apps, weather feeds, and even social media—into a single coherent operational picture. This fusion allows for earlier detection of accidents, faster validation, and more efficient emergency response. The system not only enhances situational awareness but also supports smarter infrastructure planning by highlighting patterns and bottlenecks. Ultimately, Valerann is modernising traffic management for safer, more resilient roads without the need for costly physical infrastructure expansion.
Traditional road monitoring systems often rely heavily on fixed infrastructure, which can be limited in coverage and responsiveness. The increase of data sources (CAV, crowdsourced data, navigation apps etc) brings an opportunity to enrich situational awareness of TMCs; but it also brings a challenge of data fragmentation, data overload and confusion as deliver high volumes of unstructured data and false positives, overwhelming operators and delaying meaningful action. In response, Valerann deployed Lanternn by Valerann™, an AI-powered data fusion platform that transforms how traffic data is collected, processed, and acted upon. The key safety challenge being addressed is the speed and accuracy of incident detection. Delays in identifying road incidents can increase the risk of secondary collisions, congestion, and response inefficiency. LbV synthesises diverse data sources—floating car data, roadside sensors, navigation apps, weather feeds, and even social media—into a single coherent operational picture. This fusion allows for earlier detection of accidents, faster validation, and more efficient emergency response. The system not only enhances situational awareness but also supports smarter infrastructure planning by highlighting patterns and bottlenecks. Ultimately, Valerann is modernising traffic management for safer, more resilient roads without the need for costly physical infrastructure expansion.
Initiative date
Who was/is your target audience?
Public authorities
Fleet operators
Topic
Improve vehicles and infrastructure
Knowledge building and sharing
Provide alternative solutions
Organisation details
Valerann
Enterprise
Spain
Girona
Contact name
Olga Gonzalez
Telephone number
+34657791871
olga.gonzalez@valerann.com
Website link
Project activities
If you work together with external partners, list the most important partners and briefly describe their role.
This project was made possible through close collaboration between three core partners: Valerann, Transport Infrastructure Ireland (TII), and Roughan & O’Donovan (ROD). TII, Ireland’s national road authority, led the initiative by setting the strategic vision and providing access to the national road network—specifically the M1 and M6 motorways—for testing and implementation. TII played a central role in defining project goals, such as faster incident detection, improved operator efficiency, and smarter infrastructure planning. They also oversaw performance validation and ensured alignment with national traffic and sustainability objectives.
Roughan & O’Donovan (ROD), a leading Irish engineering consultancy, brought deep domain expertise in traffic operations and intelligent transport systems (ITS). ROD supported the design and implementation of the system and provided critical insights into adapting the technology to local conditions and regulatory standards. Their role included acting as an engineering and operational bridge between TII and Valerann.
Valerann, as the technology provider, deployed and configured Lanternn by Valerann™, flagship AI-powered data fusion platform. Valerann were responsible for integrating multiple data sources, calibrating detection algorithms, and delivering actionable insights to control centre operators. The synergy between these three organisations was essential to the project’s success.
Roughan & O’Donovan (ROD), a leading Irish engineering consultancy, brought deep domain expertise in traffic operations and intelligent transport systems (ITS). ROD supported the design and implementation of the system and provided critical insights into adapting the technology to local conditions and regulatory standards. Their role included acting as an engineering and operational bridge between TII and Valerann.
Valerann, as the technology provider, deployed and configured Lanternn by Valerann™, flagship AI-powered data fusion platform. Valerann were responsible for integrating multiple data sources, calibrating detection algorithms, and delivering actionable insights to control centre operators. The synergy between these three organisations was essential to the project’s success.
Please describe the project activities you carried/are carrying out and the time period over which these were implemented.
The project began as part of a six-year strategic shift by Transport Infrastructure Ireland (TII) toward intelligent, data-driven traffic management. Working alongside TII and engineering consultancy Roughan & O’Donovan (ROD), Valerann deployed its AI-driven traffic monitoring platform, Lanternn by Valerann™, to enhance operational safety and efficiency on the M1 (with moderate ITS coverage) and M6 (with lower level ITS coverage) motorways. These routes are critical national corridors with varying levels of ITS infrastructure. The core activity involved integrating LbV into TII’s operational environment to process and analyse real-time and historical traffic data. For three months, the system operated in parallel with existing control centre tools to measure its performance. During this time, LbV proved its effectiveness by identifying incidents significantly earlier than the traditional systems—up to 35 minutes faster on the M6—and condensing over a hundred alerts into a single, actionable incident. It also surfaced up to 20 additional incidents per day that otherwise went undetected. The platform not only improved detection rates but also reduced noise, helping traffic operators focus on meaningful events. Beyond this initial phase, the system’s insights are now contributing to long-term ITS planning and investment decisions, laying the groundwork for scalable and sustainable traffic management across Ireland’s road network.
In terms of implementation, what worked well and what challenges did you need to overcome?
The project implementation benefited greatly from strong collaboration between Valerann, TII, and ROD. A major success was the seamless integration with existing ITS infrastructure and other data sources. Despite the challenge of working across motorways with different levels of ITS coverage, the platform consistently delivered strong results. What worked particularly well was the AI system’s ability to drastically reduce incident detection time—by up to 35 minutes on the M6—while also filtering out false positives, allowing operators to focus on verified, high-confidence events. The cloud-based and modular nature of LbV allowed it to adapt to Ireland’s unique operational environment without requiring extensive hardware upgrades. However, the project was not without challenges. Integrating diverse data sources—including floating car data, navigation apps, and roadside sensors—required meticulous calibration to ensure data quality and reliability. Change management within control centres was another hurdle; operators needed to trust and adopt a new AI-driven system alongside legacy tools. These challenges were overcome through continuous user engagement, transparent performance assessments, and iterative improvements to the platform. Ultimately, the project proved that data fusion and AI can significantly enhance traffic operations, even in complex, real-world environments with varying infrastructure maturity.
Evaluation
Please summarise how you have evaluated the initiative’s impact (e.g. social media reach, survey, feedback forms, statistics).
The impact of the initiative was evaluated through a rigorous three-month assessment comparing Lanternn by Valerann™ (LbV) against Transport Infrastructure Ireland’s (TII) existing traffic management systems. LbV was deployed in parallel with current control centre tools on the M1 and M6 motorways, enabling direct performance comparisons. Key metrics included incident detection speed, detection rates, false positives, and system responsiveness. Quantitative analysis showed LbV significantly outperformed traditional detection methods. On the M1, where moderate ITS infrastructure exists, 47% of incidents were identified by LbV an average of 25 minutes earlier than the control centre. On the M6, with lower ITS coverage, 65% of events were detected 35 minutes faster.
One standout incident involved a multi-vehicle collision on the M1, which LbV detected in under one minute—compressing 134 separate alerts into one actionable report.
Additionally, LbV surfaced extra actionable traffic events per day that went entirely undetected by the Traffic Management Centre (TMC). If extrapolated to the country-wide scale, this would equate up to 20 incidents per day. These were incidents requiring operational attention but missed by legacy systems due to data gaps or alert fatigue. The reduction in false positives and noise further highlighted LbV’s operational value, offering a high-confidence alert environment that greatly improved situational awareness and operator efficiency.
One standout incident involved a multi-vehicle collision on the M1, which LbV detected in under one minute—compressing 134 separate alerts into one actionable report.
Additionally, LbV surfaced extra actionable traffic events per day that went entirely undetected by the Traffic Management Centre (TMC). If extrapolated to the country-wide scale, this would equate up to 20 incidents per day. These were incidents requiring operational attention but missed by legacy systems due to data gaps or alert fatigue. The reduction in false positives and noise further highlighted LbV’s operational value, offering a high-confidence alert environment that greatly improved situational awareness and operator efficiency.
What has been the effect of the activities?
The initiative has had a substantial impact on road safety, incident management, and operational efficiency. By deploying Lanternn by Valerann™ (LbV), TII and its partners introduced a new way in how traffic events are detected, validated, and responded to. The most immediate effect was a dramatic reduction in incident detection time—up to 35 minutes faster on the M6 motorway and 25 minutes faster on the M1. This earlier detection enabled more rapid emergency responses, reducing the likelihood of secondary collisions and minimising congestion-related delays. The project also enhanced traffic operators’ ability to prioritise incidents effectively. LbV condensed large volumes of raw sensor and data stream inputs—sometimes over 100 separate alerts—into single, clear, context-rich events. This greatly reduced cognitive load for operators and improved the accuracy and speed of their interventions. Perhaps most notably, the system identified additional daily traffic events (20, if scaled to the entire country of Ireland) that had gone unnoticed by the existing control systems. These included slowdowns, breakdowns, and minor incidents that, while not catastrophic, posed risks to traffic flow and road user safety. Overall, the activities introduced a proactive, intelligence-led approach to road management that improved safety outcomes, optimised resources, and laid the foundation for long-term ITS strategy in Ireland.
Please briefly explain why your initiative is a good example of improving road safety.
This initiative exemplifies how innovative technology can directly and measurably improve road safety. Traditional road safety systems often suffer from blind spots, especially in areas with sparse ITS infrastructure, leading to delayed or missed incident detection. Lanternn by Valerann™ (LbV) overcame these limitations by fusing real-time data from multiple sources—such as GPS, sensors, apps, and weather services—into one accurate and unified traffic picture. A compelling illustration of its impact was a multi-vehicle crash on the M1 motorway. While the traditional Traffic Management Centre (TMC) required significant time to recognise the incident due to fragmented alerts, LbV detected and consolidated 134 disparate alerts into a single, high-confidence alert within one minute. More broadly, the platform identified additional daily incidents that required operator’s attention (up to 20 actionable events per day) that were completely missed by the TMC, often due to insufficient ITS infrastructure coverage or operator overload. These included breakdowns and sudden slowdowns that posed immediate but previously undetected safety risks. By reducing detection time and highlighting overlooked incidents, LbV enabled faster emergency response and mitigated the risk of secondary accidents. The project demonstrates that modern, AI-enabled systems can close critical gaps in traditional road safety operations, making roads safer without the need for expensive physical infrastructure expansion.
How have you shared information about your project and its results?
Yes, we and TII have shared these results with European public authorities (CEDR), industry associations (IBTTA, ASECAP, IRF), general and the transportation industry press.
Supporting materials
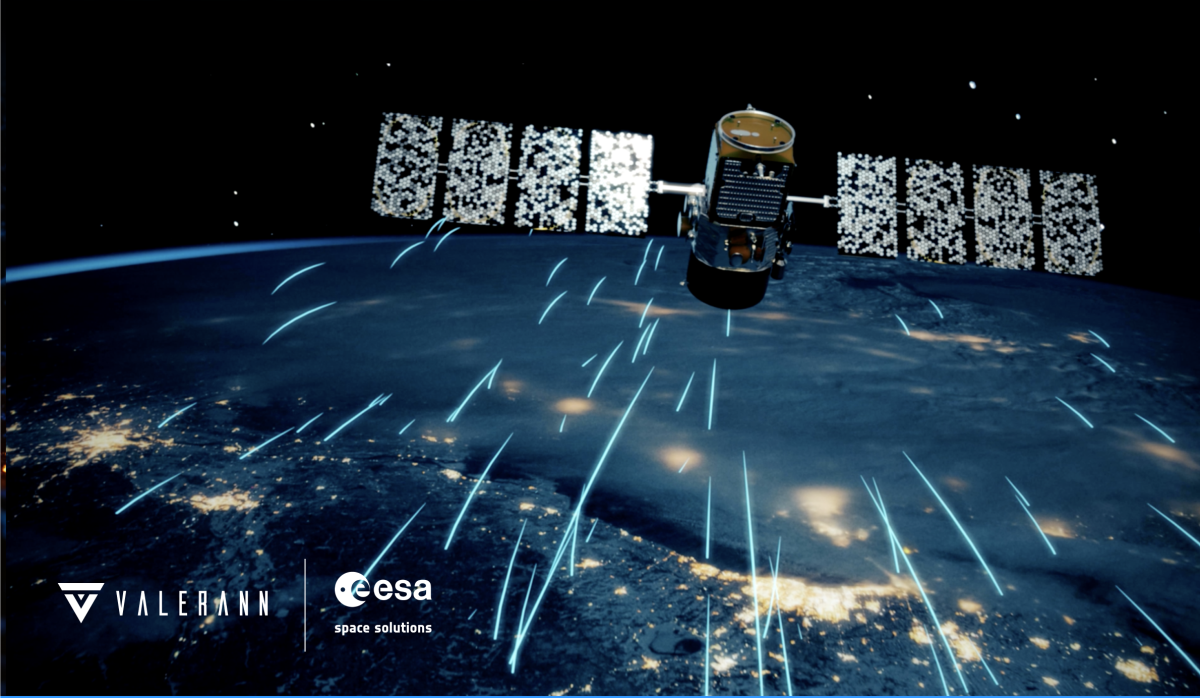